About me
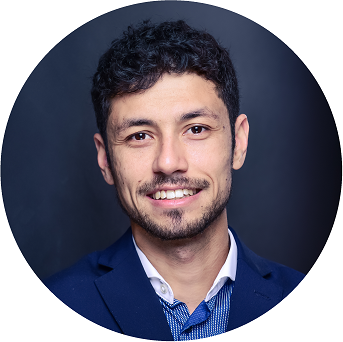
Rodrigo da Silva Alves
Currently, I am an Assistant Professor (Recombee Lab's member) at the Department of Applied Mathematics, Faculty of Information Technology / Czech Technical University (FIT/CTU). My research focuses on recommender systems (theory and applications) and, more recently, sports analytics. However, I am interested in machine learning in general, especially related to data mining and how artificial intelligence correlates to other areas of computer science. Previously, I completed a Ph.D. in Computer Science at the Machine Learning Group under the supervision of Prof. Marius Kloft at TU Kaiserslautern , Germany. I hold a Bachelor's degree in Information Systems and a Master's in Computer Science from the Department of Computer Science at the Federal University of Minas Gerais under the supervision of Prof. Renato Assunção. I am also a vocational educational teacher certificated by the Häme University of Applied Sciences, Finland, and I was a Lecturer from the Department of Applied Social Sciences at CEFET-MG. During my career, I had the pleasure of collaborating with various research groups (in different countries), outstanding students, and technical teams.
My hometown is Belo Horizonte (you can also say Beagá), Minas Gerais, Brazil. I am a Cruzeiro Esporte Clube fan and a enthusiast of Brazilian (multi-)culture and music, particularly Bossa Nova. I believe in the need of building an inclusive, accessible, multicultural, and prejudice-free environment for research.
Resume
Professional Experience
Assistant Professor (Odborný asistent)
Feb 2022 - present
Department of Applied Mathematics
Czech Technical University in Prague
Prague, Czech Republic
Researcher (Wissenschaftlicher Mitarbeiter)
Jan 2019 - Jan 2022
Machine Learning Group
Technical University of Kaiserslautern
Kaiserslautern, Rhineland-Palatinate, Germany
Lecturer (Professor EBTT)
Apr 2014 - Dec 2021 (On Ph.D. leave from Feb 2018)
Department of Applied Social Sciences
Centro Federal de Educação Tecnológica de Minas Gerais
Belo Horizonte, Minas Gerais, Brazil
Education
Dr. Rer. Nat - Computer Science
Feb 2018 - Feb 2022
Department of Computer Science
Technical University of Kaiserslautern
Kaiserslautern, Rhineland-Palatinate, Germany
Thesis: Towards Comprehensive Cluster-induced Methods for Recommender Systems
Supervisor: Prof. Marius Kloft
Professional Development Program for Teachers
Apr 2016 - Dec 2016
Häme University of Applied Sciences
Hämeenlinna, Finland
Development Work: Education for the Future: Applying Student-centered Learning in Brazilian
Vocational Education
Supervisors: Dr. Essi Ryymin and Dr. Irma Kunnari
20 ECTS / 540 hours
Master of Computer Science
Feb 2013 - May 2015
Department of Computer Science
Federal University of Minas Gerais
Belo Horizonte, Minas Gerais, Brazil
Thesis: Stochastic point process mixing model for inter-event times of Web services
My Master's thesis is composed in Portuguese. Nevertheless, you may read this paper, which is an outcome of this research.
Supervisor: Prof. Renato Assunção
Bachelor of Information Systems
Feb 2009 - Dec 2012
Department of Computer Science
Federal University of Minas Gerais
Belo Horizonte, Minas Gerais, Brazil
Award: Best Student Award
News & Updates
[07/2023] 23rd European Agent Systems Summer School
Happy to announce my active participation in the 23rd European Agent Systems Summer School, hosted at the Faculty of Information Technology, Czech Technical University in Prague. For more comprehensive information, please visit the event's official website here. Additionally, you can access the slides from my presentation, which are now available here.
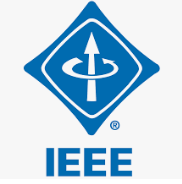
I am pleased to announce the publication of my latest academic paper on IEEE Transactions of Neural Networks and Learning Systems. The paper focuses on improving recommender systems (RSs) by addressing the issue of unequal amounts of noise in observed ratings. We propose a nuclear-norm-based matrix factorization method that leverages side information to estimate the uncertainty associated with each rating. By using this uncertainty as a weighting factor in our optimization process, we can effectively handle potentially erroneous or noisy ratings. For more details, click here.
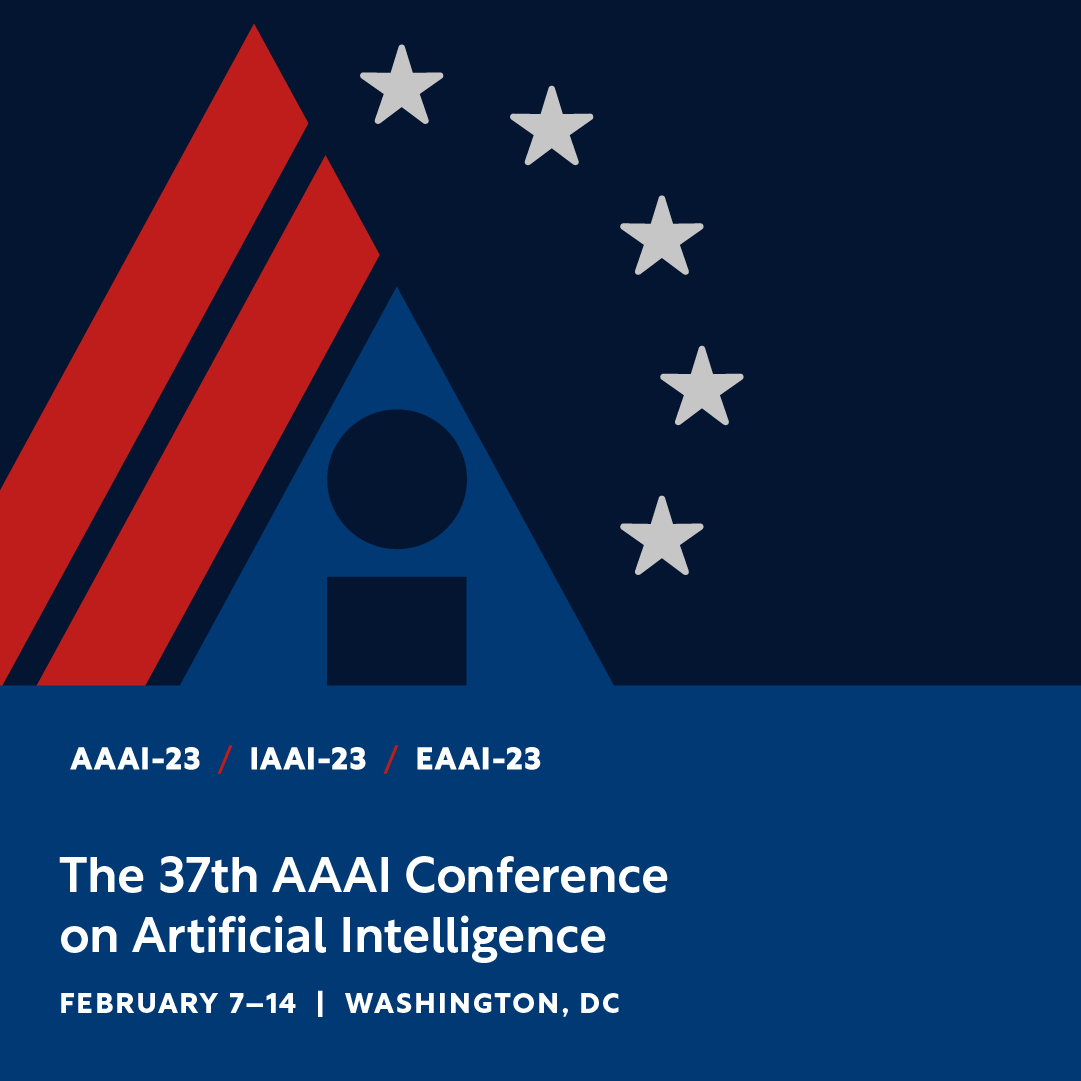
We studied inductive matrix completion (matrix completion with side information) under an i.i.d. subgaussian noise assumption at a low noise regime, with uniform sampling of the entries. The paper will appear in the AAAI 2023 proceeedings, but currently you can access here the arxiv version of the paper.
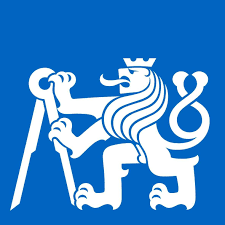
[01/2023] My website is online :)
Finally I managed to update my website. It is a working product version that will be updated time to time.
Teaching
Winter 23/24
[NIE-ML1] Machine Learning 1
[NI-PML] Personalised Machine Learning
[BI-SZ] Knowledge Engineering Seminar
Summer 22/23
[NIE-ADM] Data Mining Algorithms
[NI-ADM] Data Mining Algorithms
[NI-AML] Advanced Machine Learning
Winter 22/23
[BIE-VDZ] Data Mining
[BI-SZ] Knowledge Engineering Seminar
Summer 21/22
[NI-ADM] Data Mining Algorithms
Research
I am always open to collaborating on fascinating and challenging projects. If you are a CTU student and are looking for a Bachelor's or Master's project or would like to have your first steps in research, I would be glad to have a meeting with you and discuss the possibility of mentoring you in some projects in my research area. For internal and external collaboration, please get in touch with me by email. Below, you can find my main research contributions. Here is my google scholar profile.
IEEE Transactions on Neural Networks and Learning Systems
AAAI Conference on Artificial Intelligence
ACM Conference on Recommender Systems.
Advances in Neural Information Processing Systems
PMLR: NeurIPS Workshop on Pre-registration in Machine Learning
ACM Conference on Recommender Systems
IEEE Transactions on Neural Networks and Learning Systems
ACS The journal of physical chemistry letters
Brazilian Journal of Analytical Chemistry
ACM International Conference on Knowledge Discovery and Data Mining
ACM Transactions on Knowledge Discovery from Data
Contact
Location:
Room A-1354 / Building A, 13th floor
Thákurova 7
Prague 6 – Dejvice
160 00
Email:
rodrigo[dot]alves[at]fit[dot]cvut[dot]cz
LinkedIn:
Please do not hesitate to contact me. I am often in my office, and you can visit me without an appointment. However, I am also frequently busy, so if you want to make sure you can talk to me, send a message before. If you are a CTU student looking for projects, read about it here.
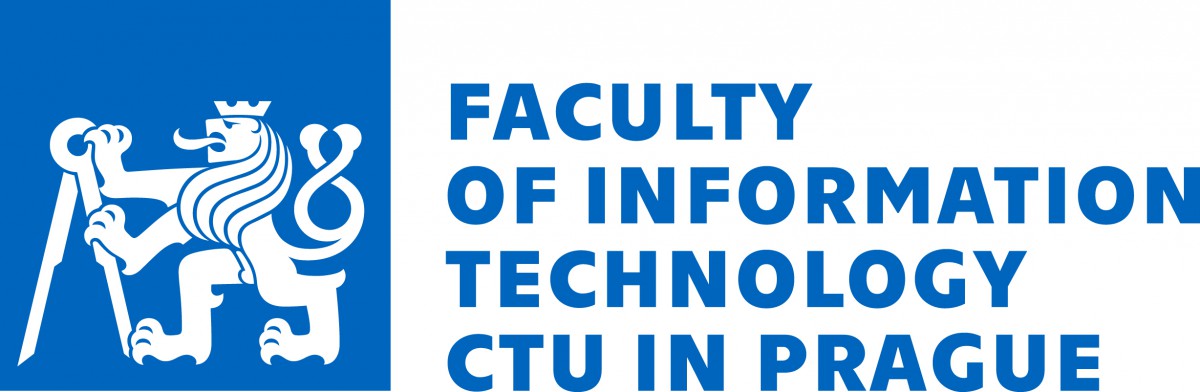